Machine Learning Models for Predicting Student Dropout—a Review
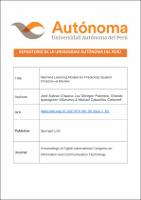
View/Open
Author(s)
Salinas-Chipana, José
Obregon-Palomino, Luz
Iparraguirre-Villanueva, Orlando
Cabanillas-Carbonell, Michael
Date
2023Metadata
Show full item recordPublisher
Springer Link
Journal
Proceedings of Eighth International Congress on Information and Communication Technology
Abstract
Student dropout is a worldwide problem that affects an entire society; thus, being of great concern for academic institutions that seek to retain their students through different strategies, machine learning is the most used for the early detection of students at risk. For this reason, in the present work, an exhaustive systematic literature review study of manuscripts related to the prediction of student dropout was carried out. The articles were obtained from six databases, which were searched using the PRISMA methodology. A total of 88 manuscripts were selected from which 4 questions were posed. Finally, we obtained as an answer to the questions that the most used model is the random forest, with an accuracy of between 73 and 99% for predicting student dropout. For this, aspects such as academic, demographic, economic, and health aspects must be considered. Meanwhile, the technological tool for the models was the Python language according to this systematic review.
Type
info:eu-repo/semantics/article
Rights
info:eu-repo/semantics/restrictedAccess
Language
eng
Collections
- Ingeniería de Sistemas [323]