Improving industrial security device detection with convolutional neural networks
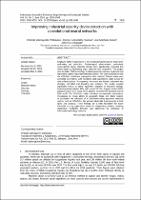
View/ Open
Author(s)
Iparraguirre-Villanueva, Orlando
Gonzales-Huaman, Josemaria
Machuca-Solano, Jose
Ruiz-Alvarado, John
Date
2023Subject
Metadata
Show full item recordPublisher
Institute of Advanced Engineering and Science (IAES)
Journal
Indonesian Journal of Electrical Engineering and Computer Science
Abstract
Employee safety is paramount in the manufacturing industry to ensure their well-being and protection. Technological advancements, particularly convolutional neural networks (CNN), have significantly enhanced this safety aspect by facilitating object detection and recognition. This project aims to utilize CNN technology to detect personal protective equipment and implement a safety implement detection system. The CNN architecture with the YOLOv5x model was employed to train a dataset. Dataset videos were converted into frames, with resolution scale adjustments made during the data collection phase. Subsequently, the dataset was labeled, underwent data cleaning, and label and bounding box revisions. The results revealed significant metrics in safety equipment detection in industrial settings. Helmet precision reached 91%, with a recall of 74%. Goggles achieved 85% precision and an 87% recall. Mask absence recorded 92% precision and an 89% recall. The YOLOv5x model exhibited commendable performance, showcasing its robust ability to accurately locate and detect objects. In conclusion, the utilization of a CNN-based safety equipment detection system, such as YOLOv5x, has yielded substantial improvements in both speed and accuracy. These findings lay a solid foundation for future industrial security applications aimed at safeguarding workers, fostering responsible workplace behavior, and optimizing the utilization of information technology resources.
Type
info:eu-repo/semantics/article
Rights
info:eu-repo/semantics/openAccess
Language
eng
Collections
- Ingeniería de Sistemas [323]