Predicting Election Results with Machine Learning—A Review
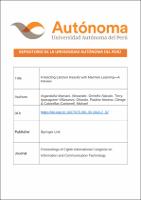
View/ Open
Author(s)
Argandoña-Mamani, Alexander
Ormeño-Alarcón, Terry
Iparraguirre-Villanueva, Orlando
Paulino-Moreno, Cleoge
Cabanillas-Carbonell, Michael
Date
2023Metadata
Show full item recordPublisher
Springer Link
Journal
Proceedings of Eighth International Congress on Information and Communication Technology
Abstract
Election results are a topic that never stops being talked about and even more so that social platforms are the perfect medium where polarization to a political party is established. That is why many academics have seen the potential of this data source for the prediction of electoral elections. Therefore, it is necessary to review what kind of machine learning models perform better in predicting election results. Therefore, a literature review is carried out, following the guidelines of the PRISMA methodology, for which databases such as Scopus, IEEE-Xplore, Science Direct, Google Academic, Springer, Ebscohost, Iop, Wiley, and Sage were used. After the literature review analysis, a total of 1638 manuscripts related to the research topic were obtained, and the inclusion and exclusion criteria were applied. Thus, 69 manuscripts were systematized. The results showed that one of the models most used by the scientific community is sentiment analysis. It was also noted that the best performing model was random forest (RF), with an accuracy rate of 97%. In the second place, we have the recurrent neural networks (RNNs) model with an accuracy rate of 91.6%. However, unlike RF, RNN requires a high computational knowledge and effort. Finally, it is concluded that the RF model is the most suitable for the prediction of electoral results since it can perform better in this type of case.
Type
info:eu-repo/semantics/article
Rights
info:eu-repo/semantics/restrictedAccess
Language
eng
Collections
- Ingeniería de Sistemas [329]