Analysis of the use of Machine Learning in the detection and prediction of hypertension in COVID 19 patients. A review of the scientific literature
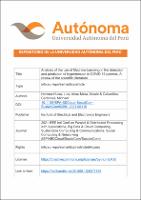
View/ Open
Author(s)
Herrera-Huisa, Luis
Arias-Meza, Nicole
Cabanillas-Carbonell, Michael
Date
2021-12-22Metadata
Show full item recordPublisher
Institute of Electrical and Electronics Engineers
Journal
2021 IEEE Intl Conf on Parallel & Distributed Processing with Applications, Big Data & Cloud Computing, Sustainable Computing & Communications, Social Computing & Networking (ISPA/BDCloud/SocialCom/SustainCom)
Additional Links
https://www.scopus.com/inward/record.uri?eid=2-s2.0-85124154461&doi=10.1109%2fISPA-BDCloud-SocialCom-SustainCom52081.202Abstract
The world is currently experiencing a major pandemic with the SARS-CoV-2 virus in which many patients who suffer and have suffered from this disease are more likely to suffer from hypertension. For this purpose, we have carried out a review of the scientific literature, from which we have collected 105 articles obtained from the following databases: ProQuest, Dialnet, ScienceDirect, Scopus, IEEE Xplore. Subsequently, based on the inclusion and exclusion criteria, 68 articles were systematized, detailing that Machine Learning helps us in the detection and prediction of hypertension in patients with coronavirus, Likewise, the predictive models that allow better detection of hypertension in patients with Covid 19 are “Neural Networks”, “Cox Risk Model”, “Random Forest” and “XGBoost”, detailing the countries and technologies used.
Type
info:eu-repo/semantics/article
Rights
info:eu-repo/semantics/restrictedAccess
Language
eng
Collections
- Ingeniería de Sistemas [323]